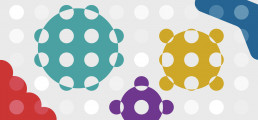
The idea of satisfying wants and needs in a mutually beneficial way is at the core of what good marketing is about. Identifying groups of customers with similar needs or preferences makes intrinsic sense to support that goal, allowing the customer experience, products, distribution, promotions et al. to be best suited to each customer. Happy customers ultimately lead to better overall results for the organisation. This classic marketing wisdom, or common sense if you like, is still as relevant now as ever.
With the ability to capture ever more data on customers, marketers and analysts alike revel in the opportunity to deliver truly personalised customer experiences. However, rising awareness about the amount of data people are sharing as part of their everyday lives has led to increased governance on what that data can be used for, and rightly so.
A recent survey by the CDEI revealed that just 51% of people are comfortable providing companies with data so that products can be tailored to them, while only 40% agree collecting and analysing data is good for society. Clearly, a sizeable portion of consumers are at odds with the very idea of parting with their data and aren’t entirely sold on what they stand to gain by doing so.
This distrust, or perhaps disillusion to certain practices, is no doubt partially product of a number of key factors, such as: the mainstream coverage of a few high-profile data breaches over the years, to the less-serious but ever-pervasive doomsday commentary around AI & automation, or simply, the sheer invasiveness of certain advertising spam that consumers are bound to encounter as part of their everyday lives.
The issue has of course not gone unnoticed, calling for some urgent action from those in and around the digital marketing industry.
Regaining trust from customers
Over the last few years, the talk of doom surrounding the reeling in of 3rd party cookies has left many acquisition-focused marketers worried about the potential impacts. The ‘phasing out’ initiative sought to prevent data collection practices allowing individual behaviour to be tracked from site to site, so that large advertising networks could target users with often spurious ads when they pop up again elsewhere (or retargeting them with a product already purchased). These tactics, deemed ‘predatory practices’, has more recently led the UK government to empower the Digital Markets Unit (DMU) to introduce new rules to help keep the likes of Google and Facebook in check and to help ‘level the playing field’ across the tech industry.
While the original intention behind some of these advertising practices may have been borne from genuine desire to target customers with the things they actually need/want, the execution became clumsy, and as noted above, often perceived as predatory to consumers and regulators alike. It was the physical equivalent of a spy following people in and out of shops, noting down what they looked at and purchased so that the spy network can show them ads at a later time.
In the physical world, that generally does not go down well and may rightfully result in a restraining order! Perhaps we could say that such a restraining order is effectively being imposed in the digital world to limit abuse of privilege and protect individual rights.
However, to continue the analogy, it is a totally different proposition if you frequent a business you like – let’s say a restaurant – and you get to know the owner a little and are happy that they know a few of your preferences. It is pretty transparent what they know, and you trust them with that. After all, it’s only which table you like and your favourite grape variety, not your deepest personal information. You are in control of the information flow.
This represents simple common sense about relationships. Trust, transparency, permission, and mutual cooperation are usually found in all of the good ones. Knowledge is built up with trust over time and helps makes things work. Of course, knowledge is power, and power is not given up lightly, hence the need for trust. This may seem like obvious stuff but guess what happens when the ad industry goes and snoops on people in attempt to find knowledge, power, and ad revenue – the trust just isn’t there.
On the other hand, if organisations treated their customers with respect and gained permissive knowledge over time, in a sensible and transparent way, customers may not fear data collection tactics but will also be providing the context and receptiveness to make insights from that data useful.
This customer data strategy fundamental is very much still in play and 3rd party cookies have never been needed to facilitate it. If you want to build long-term profitability, don’t annoy your customers – Keep it simple and honest when it comes to how you use data and how you use customer segmentation.
Customer segmentation and personalisation: Where to next?
The idealistic outcome of refining segments down to the individual level, to completely tailor experiences and offerings to customers has largely not yet come to fruition for many D2C brands. Most times, however, that level of personalisation is simply not necessary.
That does not mean that personalisation can’t still be applied or achieved, as an organisation’s approach to personalisation should be considered a spectrum of approaches rather than just a one-size-fits-all.
The granularity of an organisation’s personalisation strategy ought to be selected relevant to business requirements and objectives.
Highly sophisticated one-to-one personalisation can be potentially costly or deemed a longer-term objective due to the need for enhanced data collection and/or systems integration. Also, as noted above, may prove most costly in terms of how you are perceived by your customers. Conversely, basic customer segmentation may be considered immature or ineffective, however, fundamentally: if you are doing nothing to understand your customers then everybody loses.
Forming the right customer segmentation strategy
Leaving the often confused and misused ‘personalisation’ term aside, let’s look at how customer segmentation can be useful in a practical way based on data that is available and acceptable. We’ll focus on D2C and customer marketing scenarios in the main, but the following approaches are also valuable for a wider range of areas.
Why?
Always start by uncovering ‘the why’ – what’s the point of this exercise? what questions am I trying to answer? After all, there are hundreds of scenarios where breaking a large group into smaller segments can be beneficial.
You might be looking to understand your customer base better, define and reach new audiences, validate previous work on personas / segments, dig into campaign or conversion performance to find optimisation opportunities, or perhaps to support customer LTV decisions. Being clear about your aim will help to frame the objectives of your customer segmentation strategy.
What?
Look at what data you already have – you might not know what your customer had for breakfast this morning or what they are thinking right now, but if you’re an established company, you will have some transactional data as a minimum.
Transactional data can be used to profile your customers by when they buy, the channel they buy through, the value they bring and even the longevity of their relationship with the brand. Additional insight can then be gained by overlaying other factors such as geography, demographics, and general usage patterns, bringing your customers even further to life.
Depending on your project, you may also want to combine that data with some fresh research data, either to enhance your ability to find meaningful segments or to augment the segment profiles that you will be working with. Quantitative research data will be easier to combine with in-house behavioural data for practical analysis, but there are also ways to use qualitative data too within the same project to add depth – especially to qualify understanding of motivations and purchase rationale.
How?
There are some sophisticated approaches to help with ‘how’ or the approach, like various clustering algorithms (which will be discussed later) but there are also some much simpler techniques. In fact, often, experienced Data Scientists will begin exploring data with simple techniques before going on to apply clustering algorithms.
Once you’ve established what data is useful (e.g., how complete it is, etc), have done some data clean-up and preparation, you start looking at some key relationships between data variables and see what shapes and groupings are starting to emerge.
You also need to think about how many segments you want to end up with. Around six is a good rule of thumb in terms of usefulness, depending on the application. Of course, there will be some push and pull between your ideal number of segments to work with and what is sensible based on the data you are seeing.
This is not the place for an in-depth review of the many techniques that can be applied to segment data, but to paint the picture a little, in the section below are a few commonly used ones. These come in the form of techniques that are simple to understand and execute, through to those that are more complex, including supervised and unsupervised machine learning.
It’s very much context dependent, but we do like to use a mixture of supervised and unsupervised learning where possible, as both have benefits. Unsupervised lets the data show you the patterns rather than any biases potentially leading the way (although bias can clearly occur in the data prep stage too). While supervised techniques allow you to adjust somewhat for business needs or realities.
A few common methods of segmentation analysis:
Example technique types:
- Correlation/Bivariate analysis
- Factor Analysis
- Principal Components Analysis
How it works:
Exploring your data to spot quick insights and simple to use segments that can be quickly and easily deployed across a number of areas.
Tips and considerations for its application:
Can be used as standalone methods or as a first step to ML driven clustering methods.
Done well this step can provide the understanding necessary to get the best out of segments created.
Example technique types:
Rules-based segmentation based on specific marketing criteria
- Recency
- Frequency
- Monetary Value
How it works:
Creates groupings by looking at combinations of Recency, Frequency and Monetary Value dimensions.
These are typically very practical dimensions to work with when setting a business framework for customer strategy.
Tips and considerations for its application:
Spending time exploring the data to create bandings that work for you is essential to a successful RFM model.
Variations of these metrics can be explored to build similar models e.g., engagement rather than monetary transactions.
Monitoring and tracking these changes over time, or customer movements between segments can both inform marketing strategy as well as monitor the success of initiatives in these areas.
Example technique types:
Unsupervised Machine learning models such as
- K-means
- DBScan
How it works:
Multiple variables are fed into an algorithm that can determine sensible clusters or groups having similar characteristics within the cluster.
Clustering algorithms are often split into the following categories
- Centroid-based
- Density-based
- Hierarchical-based
- Distribution-based
Tips and considerations for its application:
Align the model inputs with the business objectives to ensure the most actionable segments are generated. Different models can be generated and operate well in parallel with each, e.g.
- Demographic/Persona generated clusters
- Behavioural clusters
- Product/transactional clusters.
Hybrid modelling also works well with segmentation, so applying PCA, for example, before inputting data into a K-means model or applying two-step algorithms can vastly improve the outputs.
Example technique types:
Deep Learning clustering algorithms such as Kohonen networks
How it works:
Like k-means clustering, this technique attempts to find groups with similarities, but works fundamentally differently by utilising ANNs to map the data into clusters.
Tips and considerations for its application:
SOMs can work great with data that has high dimensionality and is a great tool to use alongside other methods of data exploration for determining naturally occurring clusters in your data.
Example technique types:
Supervised ML algorithm
How it works:
Decision trees are typically used for classification problems however with some adjustments the outputs can be utilised to build simple and easy-to-use segments from your data.
Tips and considerations for its application:
Decision Trees can work great for developing segments with a very focussed target e.g., targeting customers who are most likely to respond to a campaign or who are most likely to convert online. The ease of interpreting the rules from a decision tree can aid in developing some core rules-based segments/audiences for specific objectives.
Closing thoughts
Using data appropriately for customer segmentation is beneficial for both the organisation and its customers – and based on the 40% who believe in the benefits to society, there is a desire to embrace the advantages that data can bring to our lives. It is essential however that we strive to see through the eyes of our customers and consumers through the entire process of collecting, analysing and implementation of solutions. As we looked at above, the rather opaque use of 3rd party cookies in recent years has done much to damage the appetite for the collection and processing of consumer data. Layer in the influence that large tech companies have had in battling to own the control and rights for our own data, it’s not surprising that the 60% still need convincing. Too little consideration was given to longer-term impact in consumer trust and respect.
However, there is still a necessary and strong role for data-led customer segmentation in serving our customers well and making their lives better. We’ve looked briefly at a variety of practical approaches to provide segmentation at the right time and in an appropriate context. However, the overarching framework for delivering a successful customer segmentation lies in the true desire understand our customers’ needs in order to serve them better.
To speak to a member of our team about any of the themes discussed in this blog, including data collection, customer segmentation, and data strategy, feel free to contact us. And to stay up-to-date with all of our latest news, including future blog updates from the team, be sure to follow us on Twitter and LinkedIn.
About the author
Anne Sargeant
With 20+ years of analytical experience, Anne Sargeant heads up Lynchpin’s Data Science team.
Anne has a degree in Maths and Physics and a master’s degree in Statistics. She is an expert in data mining, predictive analytics, and statistical modelling – with a passion for driving business success from their effective deployment.
Prior to joining Lynchpin in 2013, Anne worked as a senior analyst for Experian, Symantec, Avis and SPSS.